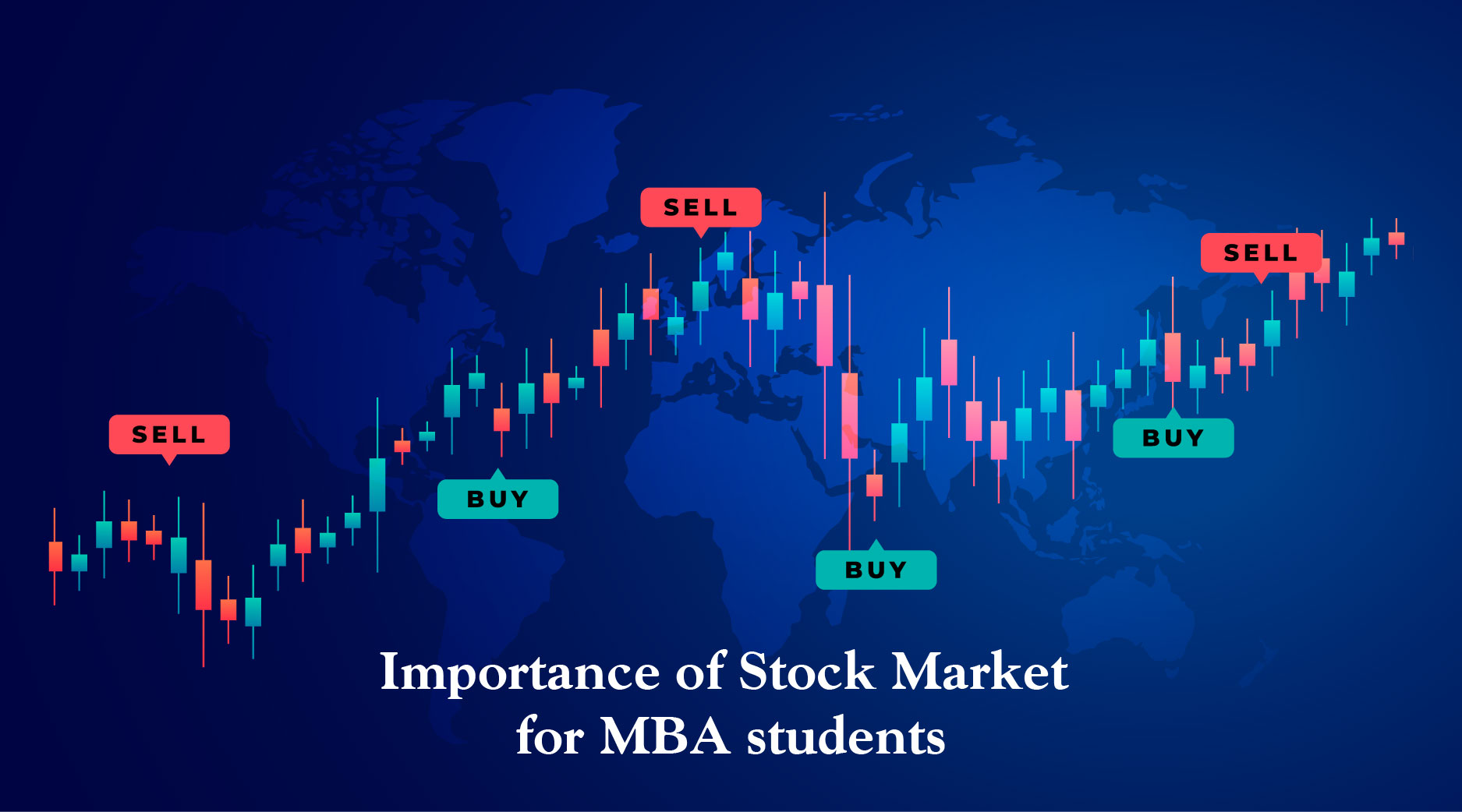
Stock Exchange Prediction Analysis
Stock exchange prediction analysis is the process of using data analytical tools and techniques to predict the future performance of stocks or other securities traded on a stock exchange. This can be done by analysing a variety of data sources, such as financial statements, market data, and economic indicators, and using statistical techniques and machine learning algorithms to identify patterns and trends that can help inform predictions about future stock performance.
There are many different approaches to stock exchange prediction analysis, and the specific methods used will depend on the goals of the analysis and the data available. Some common techniques include:
Fundamental analysis: This involves analysing a company's financial statements, such as its income statement, balance sheet, and statement of cash flows, to determine its financial health and future growth prospects.
Technical analysis: This involves analysing historical price and volume data for a stock or other security to identify patterns and trends that can inform predictions about future performance.
Sentiment analysis: This involves analysing news articles, social media posts, and other sources of information to gauge the overall sentiment towards a particular stock or market.
Recently there has been a rise in the use of machine learning for stock exchange prediction analysis. Machine learning is a subset of artificial intelligence that involves the use of algorithms and statistical models to enable computers to learn and improve their performance over time without being explicitly programmed. In the context of stock exchange prediction analysis, machine learning can be used to improve the accuracy and efficiency of predictions by enabling computers to learn from historical data and identify patterns and trends that may not be apparent to human analysts.
Here are a few examples of how machine learning can be used to extend stock exchange prediction analysis:
Predictive modelling: Machine learning algorithms can be used to build predictive models that can analyse historical data and make predictions about future stock performance. These models can be trained on a variety of data sources, such as financial statements, market data, and economic indicators, and can use techniques such as regression analysis and decision trees to make predictions.
Sentiment analysis: Machine learning algorithms can be used to analyse news articles, social media posts, and other sources of information to gauge the overall sentiment towards a particular stock or market. This can help inform predictions about future performance.
Trading systems: Machine learning algorithms can be used to build automated trading systems that can analyse market data in real-time and make trades based on predefined rules or strategies. These systems can be trained on historical data and can adapt to changing market conditions over time.
Why should MBA students study stock exchange prediction analysis?
Many MBA students are interested in pursuing careers in finance or investment, and knowledge of stock exchange prediction analysis can be a valuable asset in these fields. Understanding how to analyse and predict stock performance can help MBA students gain a competitive edge when seeking job opportunities in the finance industry.
Studying stock exchange prediction analysis can help MBA students gain a better understanding of the stock market and the factors that can impact stock performance. This can be useful for MBA students who are interested in investing their own money or who may be called upon to make investment decisions on behalf of others.